KU lab (Research)
Our lab’s research philosophies are: (1) develop game-changing technologies, (2) be genuine and respectful, and earn respect from our peers, and (3) enjoy doing it. More specifically, we wish to be the first penguin and run triumphally with big smiles on our faces. We identify critical paths for the next quantum leap. We then develop technologies that are technically/scientifically sound and work well and robustly in the real world. Currently we are focusing on two research areas—(A) spectral, photon counting detector CT and (B) intra-intervention soft tissue perfusion using x-ray angio images—while there are other topics we have seed ideas and wish to take on.
- Spectral, photon counting detector (PCD-)CT
Spectral PCD-CT acquires 3-D “tensor” information, whereas the current CT measures 3-D scalar information [read our essay]. As we envisioned back in 2013 [read our Vision 20/20 paper], four core research areas for PCD-CT are detectors/ASIC, algorithm/modeling, system designs, and clinical applications, and we are working on the topics outlined below. Projects are funded by NIH, Siemens, and Canon. This is an emerging field with many interesting topics to explore; we are looking for new industry and academic collaborators.
- Algorithms
We are developing algorithms to achieve three goals, and we are one of pioneers of this field: (1) to compensate for imperfect PCDs, (2) to handle characteristics unique to PCDs, and (3) to take an advantage of spectral information PCD provides. An example of (1) is spectral distortion compensation algorithms. As outlined below, PCDs are not flawless, and if left uncorrected, distorted spectra would result in biases and artifacts. Our PCP algorithms integrate a PCD model into the forward imaging chain and compensate for the effect during the image reconstruction process. This approach puts the complex PCD flaws into a well-posed problem framework such that we can offset the effect [see this paper, this, and this]. An example of (3) is joint estimation of tissue types and attenuation maps [see this paper]. Instead of performing multiple steps in image recon, image segmentation, and tissue type analysis, we propose to perform them jointly, and hence, to improve the accuracy of each step. We believe that spectral PCD-CT provides us so many opportunities to explore, and we started scratching the surface.
Forward imaging chain with PCD model used in a PCD compensation algorithm Spectral distortion compensation (PCP) algorithm had practically no biases Joint estimation maximum a posteriori (JE–MAP) estimates tissue types and CT images from PCD projections JE–MAP maintains the image sharpness while decreasing noise significantly
- Physics and detector modeling
We are proud to be the front runner of this effort. PCDs are not flawless due to imperfect crystals, detection physics, limited detector speed, and detection schemes implemented. It is critical to have a good detector model for studying PCD data, developing algorithms that work well with actual PCDs, evaluating performances, designing detectors, etc. The model has to be simple enough to be tractable but also accurate enough to be practically useful. More than 15 years ago, at the beginning of our PCD-CT work, we decided to take on this challenge for the algorithm development, and we have successfully modeled most of critical phenomena such as charge sharing, pulse pileup, energy–voltage response, and electronic noise. We made our Photon Counting Toolkit program (PcTK, pctk.jhu.edu) available to academic researchers in 2018 and have released more than 85 licenses to the entire world. See this paper, this, and this.
- Detector designs
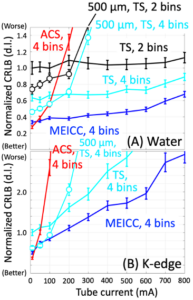
Hardware is not our strengths, but when we get an intriguing idea, we should work on it. While listening to Scott S. Hsieh’s presentation at a workshop and discussing it with him after the session, an idea of a new photon detection scheme occurred to Ken, which was later named MEICC for multi-energy inter-pixel coincidence counter. Two major problems with PCDs are charge sharing and pulse pileup; these two phenomena have conflicting characteristics, making it very difficult to solve both of them with one design. The current PCDs (TS) essentially balance them out, i.e., compromising both of them. Many advanced PCD designs such as ACS tackle charge sharing at the expense of pulse pileup problem, because they slow PCDs down. In contrast, our MEICC addresses charge sharing like a parity bit while improving pulse pileup. We believe this is a very promising technology for the next generation of PCDs and have been studying since. See this paper, this, and this.
- Intra-intervention soft tissue PErfusion assessment using x-ray angiography system with No gantry movement (IPEN)
We are developing IPEN technology to do CT perfusion, without CT. Many interventional procedures are performed to change soft tissue perfusion by, for example, removing a blood clot to recover the blood flow and embolizing arteries to kill cancers. But CT scanners are not available in intervention rooms, and we cannot assess the perfusion. We cannot tell if the procedure worked, or if we need to perform an extra procedure to complete the treatment. There is a strong clinical need, and this project aims to address it. This project is funded by NIH; we are looking for academic collaborators who will use IPEN in their studies and industry collaborators who will implement the IPEN with their angiography systems or workstations.
- Algorithms
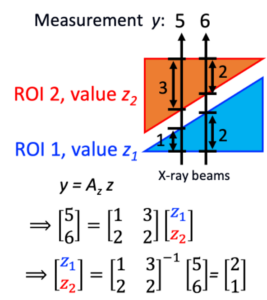
We are developing IPEN algorithms that use angiography images with no gantry/C-arm movement and estimate perfusion. It is impossible to do cross-section image reconstruction of the object from one projection obtained at one angle. But, what if the object can be divided into multiple regions-of-interest (ROIs) and the ROI shapes are known? What if each ROI has a uniform value? That is the core of IPEN algorithm. Two x-ray beams are sufficient to estimate values for 2 ROIs, right? If the object has 100 ROIs, we only need 100 x-ray beams. An x-ray detector has about a million pixels (=1,000×1,000), a lot more than 100. One projection (with 1 million x-ray beams) is sufficient to estimate 100 ROI values. Now it is a well-posed, well-conditioned problem. We are developing the IPEN algorithm for general or specific cases, when a patient moves, when only digital subtraction angiography data are available, etc.
- Brain perfusion (stroke)
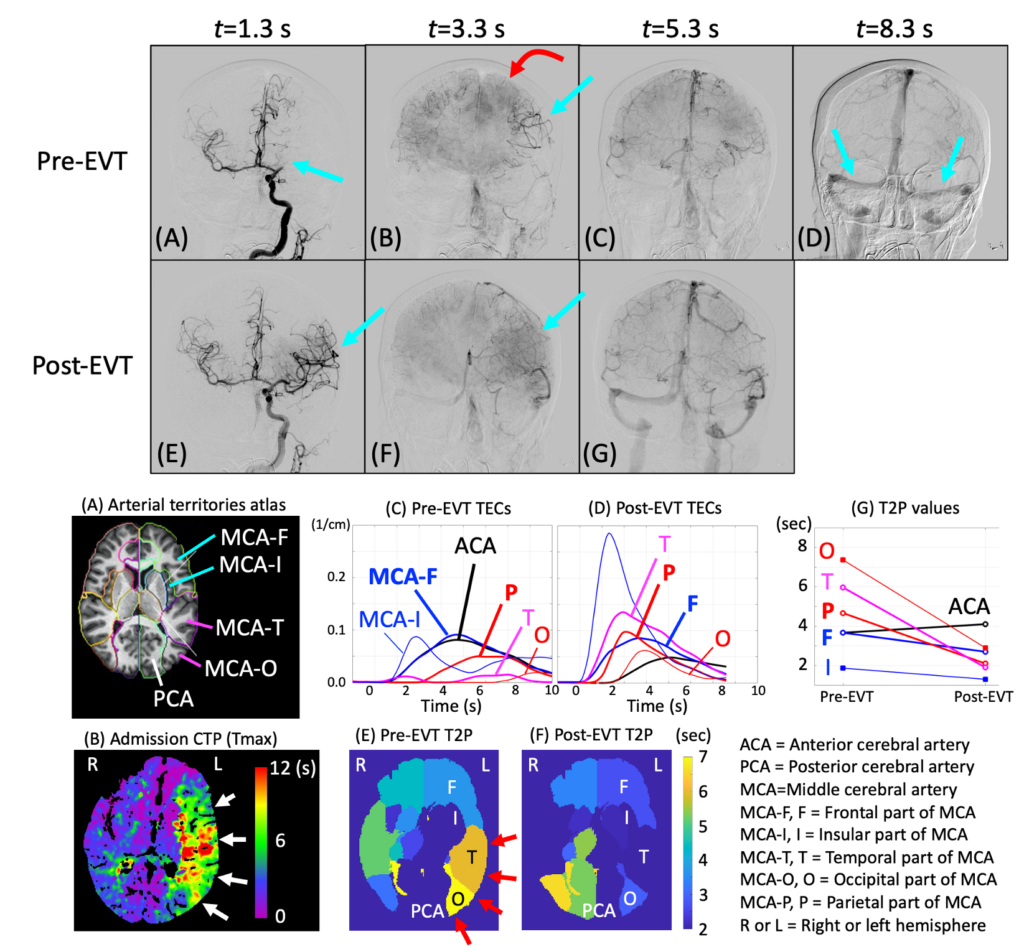
We are developing a method that uses the IPEN principle and assess brain perfusion for stroke patients. A pilot study showed very encouraging results. When fully developed, this method will consist of 6 steps and the IPEN is only 1 of the six. We are developing the other steps including head pose/orientation estimation relative to the x-ray angiography system.
- Abdomen perfusion (liver cancer)
Interventional liver cancer treatment attacks tumors by blocking the arterial blood supply and either infusing anti-cancer drug or radioactive particles. Assessment of the blood perfusion to the targeted and surrounding areas will be invaluable to maximize the treatment efficacy. Computer simulation studies show that IPEN will allow for accurate perfusion assessment [see this paper]. We plan on further development with clinical patient data.
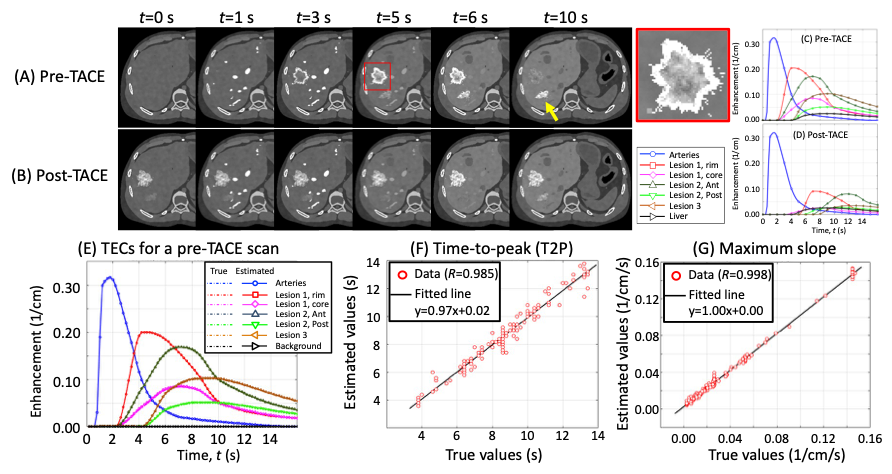
- Ischemic and infarct lesion detection for acute ischemic stroke
Our pilot study showed that non-contrast-enhanced plain head CT contains the information that is critical for scute ischemic stroke patient management. The project has been put on hold, but we would love to get back to and tackle this topic soon. See this paper.
- Motion-compensated time-resolved image reconstruction via motion estimation
This topic is where we started and we love it. It requires a blend of neat math, good engineering sense for approximation, GPU programming skills, and physiological knowledge/modeling of how the object (e.g., heart) moves and deforms. There are significant clinical merits and several interesting approaches that were only partially investigated, and one day, we would love to get back to it. See this paper, this, this, this, this, …